KGRI Start-up Research: Establishment of inexpensive and non-invasive diagnostic algorithms for dementia disease modifier
Longevity
Summary
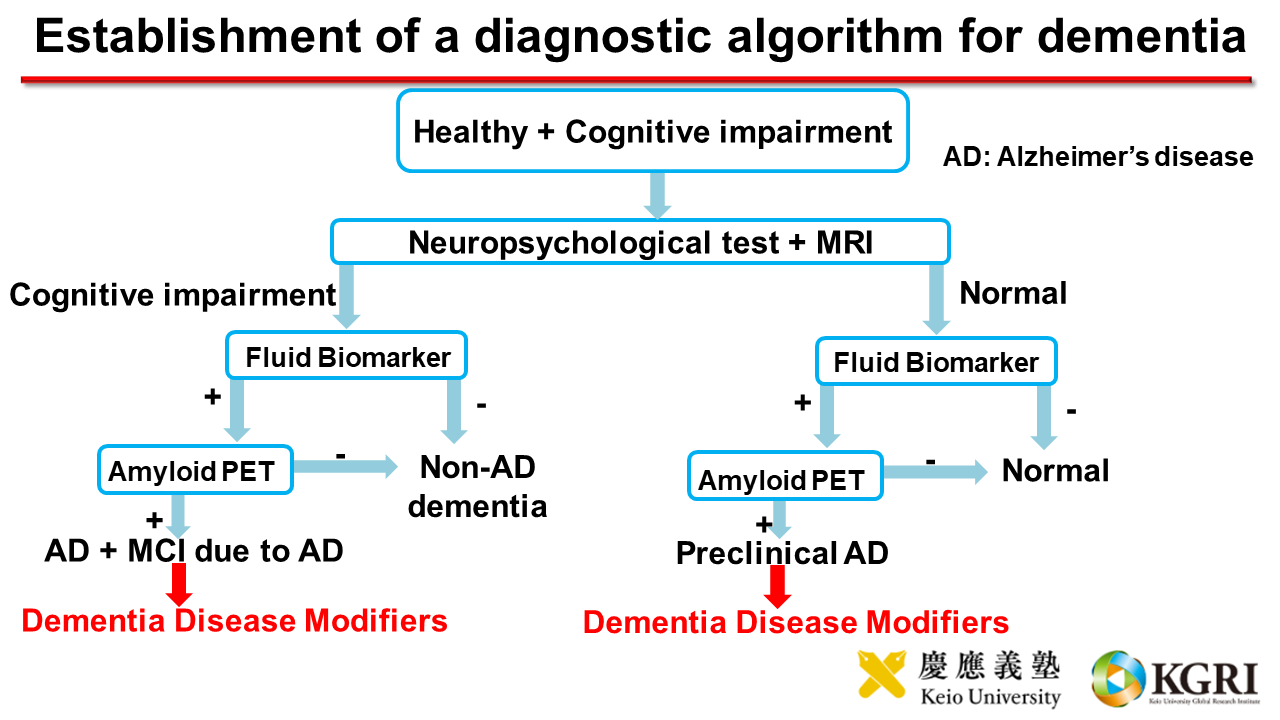
The world's population is rapidly aging, and the dementia population is projected to exceed 100 million worldwide by 2050. In Japan, the prevalence of dementia among the elderly has doubled in the last 20 years. More than half of those over 85 have dementia. Issue of dementia and a super-aging society in Japan is a World Leading Problem. The development of sustainable technologies in dementia care is considered a challenge of global social scale. It is urgent issue to develop diagnostic methods for amyloid pathology of Alzheimer's disease (AD), the most common form of dementia, and disease-modifying drugs for AD. Although there are two research level tests to diagnose Alzheimer's disease are available, amyloid positron emission tomography (PET) and amyloidβ42/40 in cerebral spinal fluid. But these are not covered by health insurance yet. Because amyloid PET is too expensive and lumber puncture is invasive, the availability of these tests is limited. In this study, we will develop a novel dementia diagnosis system by machine learning, integrating clinical information and laboratory data collected at our hospital so far. This research aims to establish inexpensive and non-invasive diagnostic algorithms for dementia disease-modifying drugs. Through 15 years of practice at our memory clinic, we have accumulated clinical and imaging data on approximately 5,000 patients with dementia. In particular, we have conducted detailed neuropsychological tests and published papers on the usefulness of these examinations. Among the above patient groups, we have clinical data on 300 cases of dementia with definite diagnosis by amyloid PET. We have also searched for noninvasive and inexpensive blood test. We found that plasma phosphorylated-tau181 (p-tau181) was significantly elevated specifically for Alzheimer's disease. We also found that combination of p-tau181 and neurofilament light chain was found to be highly sensitive in determining AD. In preliminary experiments, we have attempted to predict AD by machine learning using magnetic resonance imaging (MRI) images and neuropsychological tests. It has been confirmed that it is possible to predict AD with a correct diagnosis rate of 89.8%. Three hundred dementia patients with AD confirmed by Amyloid PET are used for training, and are trained in deep learning with Gradient-weighted Class Activation Mapping. The evaluation sample will consist of approximately 2,000 patients whose clinical diagnosis has been fixed by a 3-year cohort study. This research has led to the establishment of a novel diagnostic algorithm for dementia disease modifiers, which is for inexpensive and non-invasive diagnosis. Our simulation in this algorithm, we can estimate that a novel diagnostic algorithm can be reduced by 60% of extensive amyloid PET. The constructed diagnostic algorithm will be made widely available via the website. The effectiveness of the algorithm will be analyzed by region and compared in each country and region.
SDGs
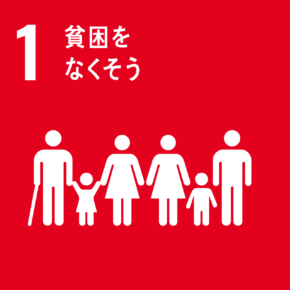
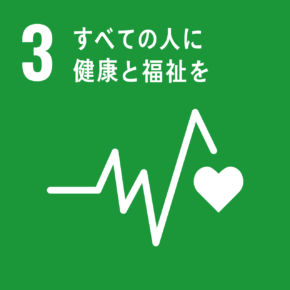
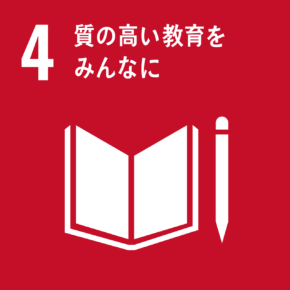
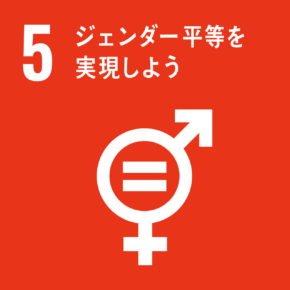
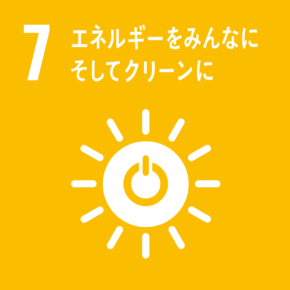
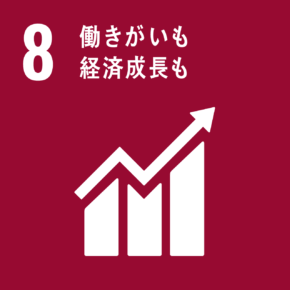
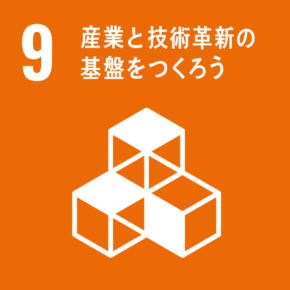
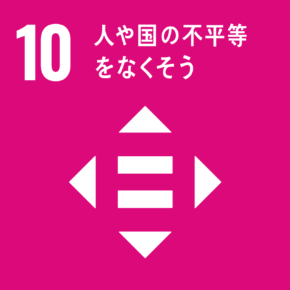
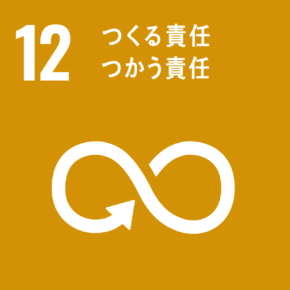
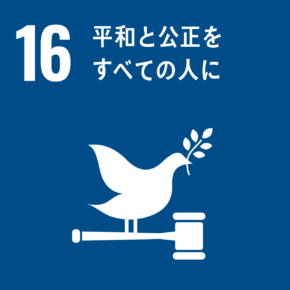
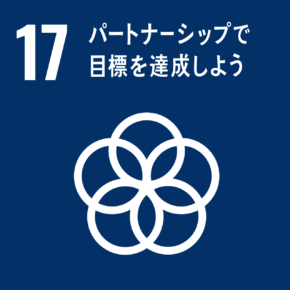
Project Members
About Project Members, ResearchersNote: ◎ indicates the project leader
Name | Affiliation | Position | Field of Specialization/Research Interests |
---|---|---|---|
◎ Toshiki Tezuka | School of Medicine | Assistant Professor | Neurodegenerative disease, Dementia, Neuroimaging |
Daisuke Ito | School of Medicine | Project Professor | Neurodegenerative disease, Dementia, Neuroscience |
Satoshi Umeda | Faculty of Letters | Professor | Cognitive neuroscience, Neuropsychology, Psychophysiology |